In a recent paper, our team demonstrated that large language models (like the ones behind ChatGPT) can be used to train algorithms that predict fraud-related securities class action lawsuits.
The study found that high-risk red flags, especially those related to corporate governance, management turnover and internal controls, often precede legal action. Hudson Labs uses artificial intelligence (LLMs) to understand the meaning of narrative disclosure in SEC filings and assess its severity.
Our study indicated that companies that were subject to a fraud-related securities class action from 2020 to 2022 (“test group”) had average risk indicator scores (based on our AI models) up to 120 percent higher than those from companies that had not been subject to a class action lawsuit since 2008 (“control group”). Details on Hudson Labs' risk indicator scores can be found in the next section.
Notably, the companies in the test group had corporate governance and management turnover risk indicator category scores that were 1.6 times higher than those from the control group. Also, the test group’s internal control risk indicator scores were 1.5 times higher than the control group.
By using the Hudson Labs Forensic Intelligence platform, you can easily identify companies with high-risk red flags related to corporate governance, internal controls, management turnover and other risks. Find a full list of Bedrock's red flag categories here. Thirteen of Hudson Labs' risk indicator categories have statistically significant associations with class action outcomes. See the complete details on our test methodology and results on our website.
Securities class action lawsuits
A securities class action lawsuits can result in significant financial losses for a company and harm its reputation. Often companies at the losing end of these class actions end up paying large settlements to their shareholders. For example, Enron Corporation paid the largest settlement of any securities class action at $7.2 billion.
The case studies section of our blog describes the machine-learned red flags previously detected by Hudson Labs at a number of companies that are currently subject to one or many fraud-related securities lawsuits:
- Coinbase Global (COIN)
- Credit Suisse (CS)
- Cano Health (CANO)
- Core Scientific (CORZQ)
- Peloton (PTON)
- Opendoor Technologies (OPEN)
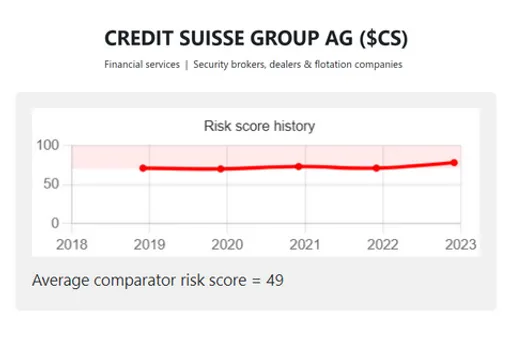
How Hudson Labs predicts fraud-related securities class actions
Our software uses large language models to identify narrative information in securities filings and assess its importance in predicting fraud. Language models facilitate a more sophisticated computational understanding of meaning. Individual sentences that have been identified by our models as noteworthy are referred to as “red flags”.
Red flags include disclosures about related party transactions, off-balance sheet arrangements, CFO resignations, and frequent changes to accounting policy, just to name a few. Because we use language models, these red flags can be detected regardless of the specific wording used in the disclosure, a significantly more robust approach compared to keyword detection techniques. Each red flag is assigned an importance score based on the sentence’s severity, according to a ranking model that takes into account the model’s confidence in its assessment.
Our models then compute “risk indicator scores”, which refer to the aggregation of total risk conveyed by all red flags within a certain category (for example, related party risk found in a company’s disclosures in the last 12 months).
We performed a statistical analysis, comparing the risk indicator scores from our test group versus those from the control group, which led to the findings discussed above.